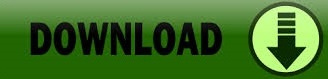
Vehicle detection methods using vehicle features, such as the Scale Invariant Feature Transform (SIFT) and Speeded Up Robust Features (SURF) methods, have been widely used. The generated optical flow field represents each pixel’s direction of motion and pixel speed. The method of using optical flow can detect the motion region in the video. Then, each frame image is compared with the background model, and the moving object can also be segmented.
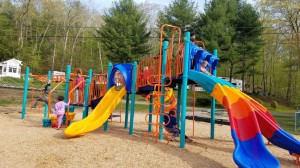
When the background image in the video is fixed, the background information is used to establish the background model. By using this method and suppressing noise, the stopping of the vehicle can also be detected. Moreover, the moving foreground region is separated by the threshold. Using the video frame difference method, the variance is calculated according to the pixel values of two or three consecutive video frames. This method can be divided into three categories : the method of using background subtraction, the method of using continuous video frame difference, and the method of using optical flow. Traditional machine vision methods use the motion of a vehicle to separate it from a fixed background image. Related work on vehicle detectionĪt present, vision-based vehicle object detection is divided into traditional machine vision methods and complex deep learning methods. In this article, we focus on the above issues to propose a viable solution, and we apply the vehicle detection results to multi-object tracking and vehicle counting. In the face of complex camera scenes, it is essential to effectively solve the above problems and further apply them. The object size of the vehicle changes greatly at this viewing angle, and the detection accuracy of a small object far away from the road is low. Generally, at a high viewing angle, a more-distant road surface can be considered. With the popular installation of traffic surveillance cameras, a vast database of traffic video footage has been obtained for analysis. Vehicle detection and statistics in highway monitoring video scenes are of considerable significance to intelligent traffic management and control of the highway. This paper has general practical significance for the management and control of highway scenes. Moreover, the novel strategy described in this article performs notably well in judging driving direction and counting vehicles. The experimental results verify that using the proposed segmentation method can provide higher detection accuracy, especially for the detection of small vehicle objects. Several highway surveillance videos based on different scenes are used to verify the proposed methods. Finally, the vehicle trajectories are obtained by the ORB algorithm, which can be used to judge the driving direction of the vehicle and obtain the number of different vehicles. Then, the above two areas are placed into the YOLOv3 network to detect the type and location of the vehicle. In the proposed vehicle detection and counting system, the highway road surface in the image is first extracted and divided into a remote area and a proximal area by a newly proposed segmentation method the method is crucial for improving vehicle detection. Compared with the existing public datasets, the proposed dataset contains annotated tiny objects in the image, which provides the complete data foundation for vehicle detection based on deep learning. A new high definition highway vehicle dataset with a total of 57,290 annotated instances in 11,129 images is published in this study. To address this issue, this paper proposes a vision-based vehicle detection and counting system. However, due to the different sizes of vehicles, their detection remains a challenge that directly affects the accuracy of vehicle counts.

Intelligent vehicle detection and counting are becoming increasingly important in the field of highway management.
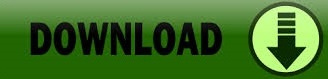